In the Age of Prediction, Algorithms, AI, and the Shifting Shadows of Risk, Tulchinsky, founder of quantitative hedge fund WorldQuant, and Mason, a geneticist and computational biologist, jointly question how the evolution of artificial intelligence, data, and prediction will play out in finance, medicine, crime
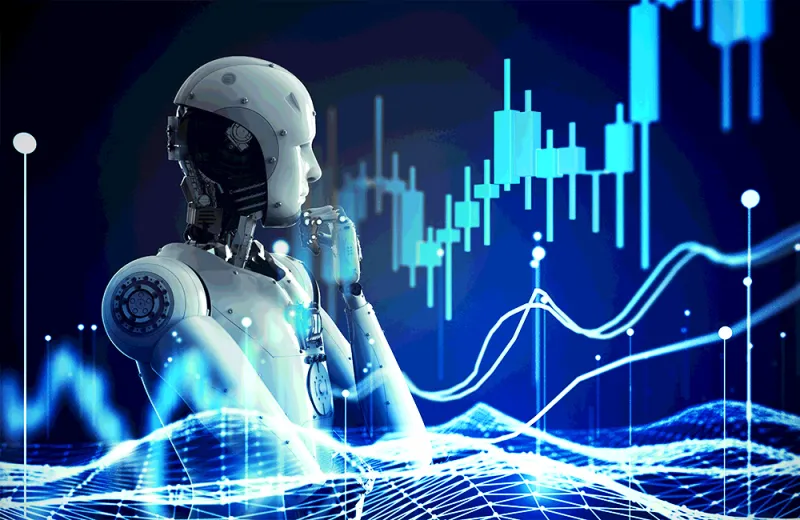
Illustration by II