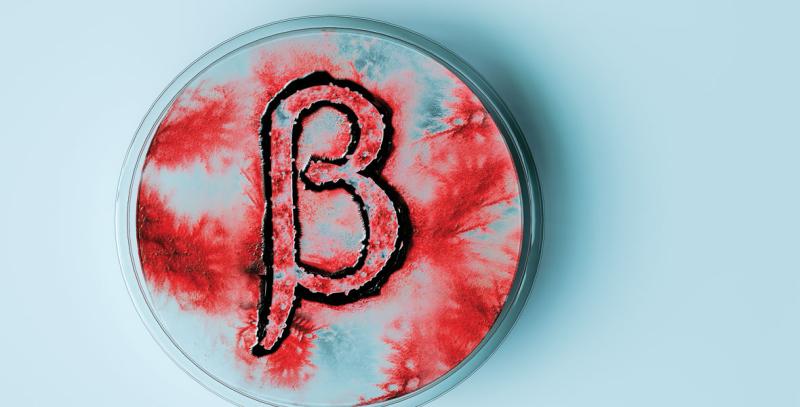
Smart beta may be state-of-the-art, but it has also become the E. coli of institutional investing. There are at least 300 published factors, with roughly 40 newly discovered factors announced each year. Industry-aligned benchmark providers like MSCI, FTSE Russell, Barclays, S&P Dow Jones, and others create mountains of research and indexes supporting these found factors. So they must be real, right? The fund management industry’s brightest idea of the past few years is to soup up index returns by ranking companies not only by size and value, but also by other properties — or factors — like quality, profitability, volatility, and dividend payouts.
If you buy into this, then you’ve determined the starting point of a conversation but botched the story and the conclusion.
Researchers Eugene Fama and Kenneth French introduced their classic three-factor model, consisting of market risk, size, and value, in 1993. Nowadays indexes are investment products in and of themselves. Firms get paid if a manager wins a mandate benchmarked to one of their proprietary indexes, so is it any surprise that these indexes are proliferating at an astonishing rate? It’s been a benchmark bonanza: EDHEC-Risk Institute’s ERI Scientific Beta offers more than 4,200 smart-beta indexes. Major index providers have become factor cheerleaders, prominent fund providers have created products based on those factors, and leading investment consultants have endorsed it all.
The sales pitch is good: Factor returns are cyclical, thus uncorrelated multifactor approaches can smooth out the dry spells. The value effect, for example, returned nada for a 20-year period, from about 1951 to 1971. In the absence of a definitive method of factor timing, the prudent approach is to diversify across multiple factors.
I get the concept. I really do. There is no Holy Grail investment style that will outperform in all market environments. And the notion of using something in combination with something else always has a good feel to it. If you love value, then you must really love value + quality. And if you like combining two risk premia, then a quad strategy must be twice as good. Factor cocktails should be more powerful than stand-alones, and blending pro-cyclical risk-on factors (e.g., value) with defensive factors (high quality, low volatility) alleviates business cycle exposure. Shake it all together — bada boom, bada bing — to reduce risk, increase diversification, and get a higher return stream. Bob’s your uncle.
Maybe, maybe not. Read on.
→ When Added up, Facts Are Lies
The virtues of diversification have been drilled into the heads of financial professionals and novices for the past 50 years. As a group, we’re primed to become enamored of the idea of capturing multiple risk premia. But that overlooks the fact that these factors often cancel each other out — they’re contrabets. It’s not easy or efficient to combine them. Adding multiple engines backed by opposing theories in a single portfolio introduces drag, because negatively correlated factor investments effectively bet against one another. Value has a well-established negative correlation to momentum: When a stock’s price declines, it becomes more “value-y,” but it also becomes antimomentum. Momentum strategies look for stocks that are going up, so a stock’s weight in a momentum-tilted portfolio will decrease at the same time that its weight in a value-tilted portfolio will increase. Combining the weightings of individual factor indexes — i.e., 50 percent momentum plus 50 percent value — will at times result in reduced exposure to both target factors.Crosscurrents abound. Quality and profitability look very similar to each other and a lot like growth, so when you add them to value, you’re moving back to neutral because value stocks more often than not are closer to the junk end of the quality-versus-junk spectrum. This is just another way of saying that value stocks are the opposite of quality stocks. Size is antimomentum. Profitability and investment are slightly long momentum, which makes them frenemies. Quality portfolios have negative market, value, and size exposures, leading this new factor to pretty much cancel out everything in the original model. We find ourselves like the White Queen in Through the Looking Glass, believing six impossible things before breakfast.
→ It’s Just More Frosting on the Same Cake
There is substantial pairwise overlap among smart-beta descriptors, so owning more than one factor is often a doubling up (crossover) of bets. Emphasizing quality companies accesses the low-volatility anomaly indirectly. In fact, adding low volatility, quality, high dividends, or profitability is pretty much the same thing. Intuitively, this makes sense: Companies with stable share prices often have the mature, steady operations that are a hallmark of regular distributors of cash. And a dividend itself can damp volatility, because the rising dividend yield that comes with a falling price can bring in buyers.The momentum factor can be something of a chameleon because it inherently chases returns wherever they may be coming from. In June 2016 the overlap of stocks between the momentum and low-volatility factor indexes reached more than 69 percent. It’s not uncommon to discover that quality, momentum, and minimum-volatility strategies are all buying the same stocks. They’re brothers from other mothers. We’re measuring the same thing twice but calling it something different, so investors trying to capture everything are overallocating to co-lineal and redundant risk factors.
Founded on colliding philosophies of investment valuation, smart beta is a modern superstition. Accounting for factors that look similar and others that cancel each other out, diversified-factor approaches look like a bowl of mush; they vary little from the broader index out of which they were created. It is analogous to plan sponsors picking active managers for every square in the Morningstar style box, and then — shock of shocks — having the composite look and behave like an index fund. Even though a total stock market fund owns both small-cap and value stocks, it has exposure to only one of the drivers of returns — beta. The total stock market fund holds small stocks but has no exposure at all to the size factor. This seeming contradiction confuses many investors. It’s true because small stocks provide a positive exposure to the size effect, whereas large stocks provide a negative exposure to it. That puts the net exposure to the size factor at zero. The same is true for value stocks, which are the opposite of growth by construction.
It’s silly, akin to double-majoring in psychology and reverse psychology. Gumming the strands of theories and countertheories into a single nut cluster isn’t a great idea because the truths recoil from each other: North negates South and East neuters West. For investors worried about market beta exposure, picking a side reduces that risk. Picking all of them invites it (Fig. 1).
Making stocks simultaneously pass all filters at once won’t work either, because individual factors’ time variation in returns differ. Momentum is like watercress: ready for harvest about 14 days after it’s sown. But value is like snap beans, which take nine or ten weeks to mature. Forcing all into one basket and rebalancing (“harvesting”) at the same time creates a whole new set of issues.
The main marketing test for these all-in-one products is academic research. Studies prove concept, and proof sells product. But few people appreciate how weak the statistical support really is. “Smart beta” is the catch-all term for funds that use statistical hypothesis inference testing (that spells SHIT, by the way), which relies on historical regressions and the resulting p-values. This approach is in the process of being discredited. A methodological crisis occurring in science has swept up investment finance as well. Researchers across disciplines have found the results of many experiments difficult or impossible to reproduce, even by the original experimenters themselves.
In 2016 the American Statistical Association published an extraordinary document. The “Statement on Statistical Significance and P-Value” reiterated that “a p-value does not provide a good measure of evidence regarding a model or hypothesis.” It cannot answer the researcher’s fundamental question: What are the odds that a hypothesis is correct? A worldwide consortium of scientists known as the Open Science Collaboration advocates abolishing the use of p-values to determine statistical significance. At least one journal has decided that it will no longer publish the metrics. It’s been a wake-up call. Most published research based on statistically significant findings is false. This is a jarring breach of faith — like a child discovering, in his father’s drawer, the Santa Claus suit.
The whole thing really started to stink when a Cornell University professor proved the existence of extra-sensory perception (ESP). That’s when a fault line opened up and the scientific community took notice. Obviously, there was something weird in the woodpile. What had gone wrong? In a word: computers. For every fact there is an infinity of hypotheses and false discoveries that grows proportionally with the number of tests. The availability of huge data sets and inexpensive computing power allowed researchers to let the PC run overnight trying thousands of combinations and report only the ones that “worked.” Sound practice dictates that you announce what you think will work and then test it. To work backward is called “p-hacking,” and it is so widespread throughout science that many published results are false positives. For example, you might p-hack to the discovery that wet roads cause rain.
→ Requiem for the Small-Cap Premium
All of this is academic, of course. Literally. I point this out because a funny thing happened on the way to the bank. Ever since the groundbreaking small-cap/size study (which had a p-value of 1 percent) was conducted at the University of Chicago and published — the factor hasn’t worked. Small-cap is the granddaddy of factor premia, but in recent decades it has been a major disappointment, lagging both in absolute returns and on a risk-adjusted basis (Fig. 2). Ken French found that the annualized mean return spread for small-caps over large-caps exceeded 7.5 percent for approximately 90 years, but it has been virtually nil since 1983. To be sure, there have been periods in which the smallest stocks have come out on top, but there has been no consistent pattern. A repeat of the 1981 study using all historical data available today would not conclude that there is a small-firm effect.The original factor models aren’t working, so some folks are trying to resurrect them by introducing new factors. The quality dimension, for example, resuscitates the small-firm effect, but we’re just plugging holes in old dissertations. The great factor debate has been overstated from the start; it was never 100 percent true because there’s very little resemblance between live long-only factor funds and the long-short academic portfolios that make the majority of their theoretical returns from shorting illiquid stocks that would be impossible to short in real life. Launched in 1978, the Russell 2000 Index is arguably the earliest factor product and the most commonly referenced U.S. small-cap index. Would it surprise you to learn that since the inception of this index through the end of 2017, a 39-year period, it has underperformed the S&P 500? So much for the small-cap effect.
It hasn’t worked in the broad scope of time, and it hasn’t worked recently. Even more damning is the markedly inferior performance of the Russell Microcap Index. Contrary to the theory, the smaller stocks have gotten, the worse they have performed. It is not complicated. The effect just is not there.
Likewise for the value effect (Fig. 3). Outside the original 1962–1981 period — during which the value premium concept was born of large-sample back tests — there is no significant evidence of a value premium. The largest investment fund with “value” in its name is the Vanguard Value Index Fund, with $44 billion in assets and, since inception 25 years ago, a losing track record to the S&P 500.
What caused the market to diverge from the classic theories? Methodological errors, to some extent, but there might be more to it. Empirical evidence has become promotional currency, derived through the biased studies of vested interests. Most of these new factors are coming from product literature, but on closer scrutiny some of the research is dodgy, some is complete bunk.
Take low volatility, for instance (Fig. 4). This relatively new theory apparently contradicts the financial tenet linking higher risk to higher returns, which is supposedly what the capital asset pricing model is all about. Is the low-volatility effect true? Well, yes and no. Mostly no. The key is selecting 1968 to begin the study. From 1929 to 1968 returns and volatility related positively. In 1968 everything reversed, and high volatility stocks began underperforming low-volatility stocks. Every result is a temporary truth
→ Confidence Tricksters
It’s a Casaubon delusion; there is no transcendent truth. P-hacking makes it easy to get plausible-sounding studies to “work,” so allow me a moment to abandon the moral high ground here and roll my eyes at the suggestion that cultural diversity really improves investment returns. The latest batch of studies by McKinsey & Co., the Clayman Institute, Credit Suisse, MSCI, and others claim that greater gender diversity at senior leadership levels leads to more perspectives, which lead to better decision-making such that companies with strong female leadership see better performance than those without. There’s an SPDR SSGA Gender Diversity Index and a SHE exchange-traded fund.I would like to acknowledge that I once thought Evelyn Waugh was a woman and that George Eliot was a man. And yes, I can see the appeal of melding a socially responsible mission — addressing the social issue of the gender gap in corporate America — with a factor-based approach, but I’m totally not buying it. The sales literature fails to note that the Pax Ellevate Global Women’s Index Fund, launched in 1993, has averaged an annual gain of 1.1 percent, below both the MSCI World Index’s average annual gain of 3.78 percent and the S&P’s average gain of 6.4 percent. Touchy topic, backlash guaranteed, but I recommend you view things as they are, not as they ought to be.
Recently, there’s been a flurry of flighty studies supporting socially responsible investing because it purportedly outperforms in the long run. I’m not sure how to reconcile such research with the fact that we all know that alcohol and cigarette stocks absolutely kill it. Tackling gender and telling people they’ve adopted an invalid belief system aren’t going to win me any friends, I recognize that. But doesn’t anybody care anymore about the integrity, transparency, and unbiasedness of science?
It pays to dig into the facts because most people care not to do so. We live in an age of “truth decay.” The Oxford English Dictionary proclaimed “post-truth” the word of the year for 2016. The original smart-beta factors aren’t working, and the science behind it is known to be flawed. But nobody’s telling the average investor, because there’s no profit in it. There is a symbiotic relationship between the fund industry and the financial media: The industry needs the media to talk about and help flog its products, and the media needs advertising revenue and something to write about.
And yes, you should be skeptical of my skepticism.
Richard Wiggins works as a strategist at a large corporate pension fund. He authored this article independent of his employer.