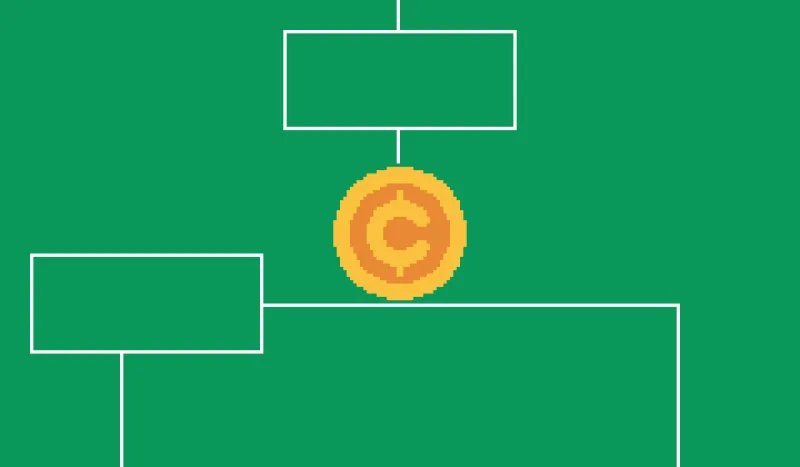
Illustration by Nick Little
On March 30, a court ruling against Goldman Sachs made Wall Street compensation consultants sit bolt upright.
In the 49-page document, U.S. District Judge Analisa Torres wrote that a group of women could proceed with a class-action lawsuit that accused the investment bank of gender discrimination regarding performance reviews, promotions — and pay. The ruling stated that the women had offered “significant proof of discriminatory disparate treatment” at Goldman.
The case itself is nothing new: The long-delayed lawsuit actually began eight years ago, and it is just one of many similar claims brought against investment banks over the past several decades. But what stood out about the Torres ruling is the timing. Over just the past three years, several states — including New York, New Jersey, and California — have passed stringent new pay-equity laws requiring companies to pay employees belonging to certain so-called protected classes, such as race or gender, the same as other employees with the same responsibilities.
The laws go further than federal equal-pay statutes in that they essentially lower the burden of proof for employees to make a credible claim. Workers simply need to show that positions are sufficiently similar and the pay isn’t. They don’t even need to have the same job title, according to Adam Zoia, a veteran executive recruiter and founder and chair of search firm Glocap Search who recently co-founded CompIQ, a software company dedicated to bringing pay into the artificial intelligence age.
“Now it’s not good enough to say we are doing the best we can,” says Zoia. “The legislation is creating liability if you don’t offer equal pay for equal work.”
Sarah Reynolds, a senior manager at Salary.com, agrees. “No one’s going to win a lawsuit by saying they never looked at their data before, so they can’t be held accountable,” she says.
And even if the reasons for pay disparities aren’t nefarious — say one employee simply negotiated harder for a higher salary — companies will still be on the hook for them.
“The compensation industry operates on imprecise data, manually analyzed,” says Zoia. “They don’t go through that for every employee; it’s too expensive. So there’s a lot of unfair pay, not for maliciousness” but because of incomplete information, he says. But the new laws don’t make exceptions for these circumstances. “This will become more of an issue as more litigation happens.”
Still, determining salaries is a thorny problem for companies, which have to factor in myriad variables, such as job responsibilities, seniority, geographic location, market competition, and even office politics. So how can companies stay on the right side of the law?
Enter the algos.
“The holy grail in comp is comparing apples to apples. But there are nuances around job responsibilities. You are comparing people at the title level with no assurance they are doing the same thing,” he explains. “It’s a well-known problem. And for people paid maximum salaries, you often don’t know why. Do they get extra money because they have been there ten years? Are they a rock star? Do they have extra responsibilities?”
That’s where AI and machine learning techniques come in. (Machine learning is the “how” and AI is the “what.” That is, AI is powered by machine learning.) Long heralded for their promise in portfolio management, AI and machine learning are now being applied to the back office. By comparing data points for salaries across numerous companies, locations, job titles, responsibilities, and so on, computers can triangulate data points to arrive at a number.
Here’s how CompIQ’s software works: Client firms upload their employees’ department, title, location, performance review, and compensation, and in a separate process, employees specify their responsibilities, which are confirmed by their managers. The data is then encrypted in transit, and machine learning models are run across this information. Clients see customized compensation benchmarking information specific to each employee.
The software is meant to automate processes Zoia describes as “manual, expensive, and inaccurate.” The tricky part, of course, is teaching the machines to recognize nuances, such as how two employees can have the same title but drastically different responsibilities.
Take analysts. There are certain baseline responsibilities, such as financial modeling, that all financial analysts can be expected to know how to do. But some have portfolio discretion, including picking stocks, which commands a higher salary in the market.
Machines are far from perfect, but letting them handle the process can help employers stay on the right side of the law. Zoia says CompIQ’s system analyzes and flags jobs where there may be pay discrepancies, a feature he expects will be offered by any competitors that enter the market.
In addition to the new pay-equity laws, several states have made it illegal for employers to ask job candidates what they made in their last jobs. Salary.com’s Reynolds says asking the question isn’t best practice anyway — but many employers still want to know.
“If you can’t ask an employee what they make now, you need an alternative way to gather that data,” she says. “Whether that’s from an aggregate data provider or a survey provider, you want to make sure you are getting valid data that isn’t influenced by an employee entering their own data on some website.”
AI systems also help with what compensation consultant Alan Johnson calls the “entitlement culture” that exists in some organizations.
“There will be charts and graphs that show, ‘Geez, Alan has been underperforming for two years — why is he paid so well?’” says Johnson. When he has flagged certain employees as being under- or overpaid, clients will acknowledge it, he says, but are often unwilling to address it due to corporate culture, politics, or an “out of sight, out of mind” attitude. AI systems “will make it harder to hide.”
Reynolds notes that such systems can also flag when superstar employees are significantly underpaid versus the market — making them a potential flight risk. Predictive analytics tools also can enable companies to identify which jobs will be in demand in the coming years, allowing them to set salary increases accordingly to stay competitive when it comes to retaining talent.
So what’s the catch with these applications?
They’re designed by humans.
For his part, Johnson is intrigued by the promise these new technologies offer, saying the field of human resources is long overdue for better analytics around pay and careers. But he wonders if the early euphoria might be overblown.
“I think it’ll be helpful, but I’m not sure it’ll be that helpful in determining the dollar amount every single person gets,” he says. “It may be a case of using a cannon to kill a flea. Many times it’s not the facts, it’s the will to do something. Do you need AI to figure it out? Sometimes you do. But it’s like Weight Watchers. We all should just eat less. But if WW or AI gets people excited to do it, then okay.”