Welcome to the world of big data. As of the middle of last year, every minute Internet users sent more than 204 million e-mails, ran 4 million searches on Google and submitted more than 275,000 new tweets, according to Domo, a software company based in American Fork, Utah (see chart). In aggregate, IBM estimates that each day the world creates more than 2.5 quintillion bytes of new data.
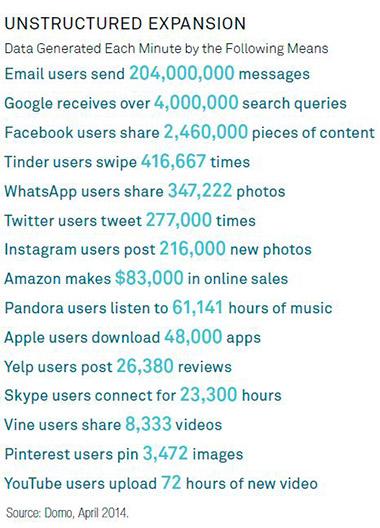
This rapid growth of often previously unavailable sets of data on a massive scale has led to the development of a new field of study at the intersection of statistics and computer science. Data science offers new tools for extracting predictive insights from enormous sets of data that had been unsuited to classic statistical models and techniques. Over the past several years, data science algorithms have become so ingrained into our daily lives, we often don’t even realize it. When we use a search engine, translate a web page to a new language at the click of a button or when our e-mail software chucks out yet another piece of spam, we are witnessing such machine-learning techniques.
Still, the cardinal sin of any statistical modeler is overfitting the data: testing and retesting model specifications to get better and better predictive power that ultimately fails when used on real-world and out-of-sample data. Researchers use a variety of techniques to avoid this danger, such as disciplined use of testing and holdout samples; a focus on predictive variables with clear sensibility, that is, being able to explain why it works; and, especially, an emphasis on simpler models with fewer free parameters to be tuned to the data in the first place. Essentially, those who manipulate data restrict their attention to models with a few variables and simple linear relations between inputs and forecasts to reduce the temptations of unfettered data mining.
The cost of these restrictions is that we lose the ability to identify some of the more subtle predictive features of these new techniques that may provide trading insights. Machine learning provides a way around that limitation, by effectively letting the data “speak” to uncover the nonlinear, or dynamically evolving, relationships across a broader set of potentially predictive variables. These techniques allow for guardrails that limit the complexity of models to their ability to forecast out of sample. Moreover, traditional statistical techniques are best suited to data sets that are organized so that there are a fixed number of fields for each observation. Machine-learning techniques, by contrast, can be applied to more unstructured data sets, like large bodies of text. Examples of unstructured data include news articles, press releases, blogs and tweets.
These algorithms and modeling techniques have been widely adopted in established industries, such as advertising and pharmaceuticals. Their adoption by asset management, however, has been much slower and less pervasive. At BlackRock, we think these new data science techniques have tremendous potential to identify and capture systematic investment opportunities for our clients, as my colleagues in our Scientific Active Equity group have recently argued. More specifically, using these machine-learning techniques, we can develop highly adaptive investment strategies that respond dynamically to evolving market conditions, we can enhance the predictive power of our trading models, and we can quantify what used to be purely subjective assessments of tone in an analyst’s report or in a CEO’s expression of optimism on a conference call.
Long-short equity research has been the natural starting point for much of this work within the quantitative finance community. This is intuitively sensible, as these big data techniques work best when they are deployed against large sets of data. Equity markets have data in abundance, with long historical records of prices across thousands of equities and volumes of writings about each firm generated each year (annual reports, analyst reviews, conference call transcripts, press releases and news articles, as well as social-media-related data like chat room and Twitter commentary).
As for fixed income, despite the generally smaller breadth of data, there are substantial opportunities to apply machine learning and big data techniques. Credit investors, like those in equities, form views on the relative health of individual issuers in the marketplace and stand to benefit from the long and short insights harvested from the growing masses of firm-specific unstructured data. More macro-focused investors stand to gain as well, as we can apply these techniques to help gauge the sentiment of relevant content, such as news articles, economic strategy research, Federal Reserve governor speeches and Fed minutes.
On a cautionary note, we should temper our enthusiasm with the recognition that modern statistical learning and unstructured data, although useful techniques to have at our disposal regardless of asset class, are not magic. Understanding market dynamics and economic insights still matters a great deal. With data science, we can use that market knowledge and investment expertise to identify and cultivate valuable data sets, guide how we apply our machine learning techniques toward harvesting predictive insights from those data and develop investment strategies accordingly.
Mike Rierson is managing director and head of research for BlackRock’s model-based fixed-income group in San Francisco.
Get more on trading and technology.