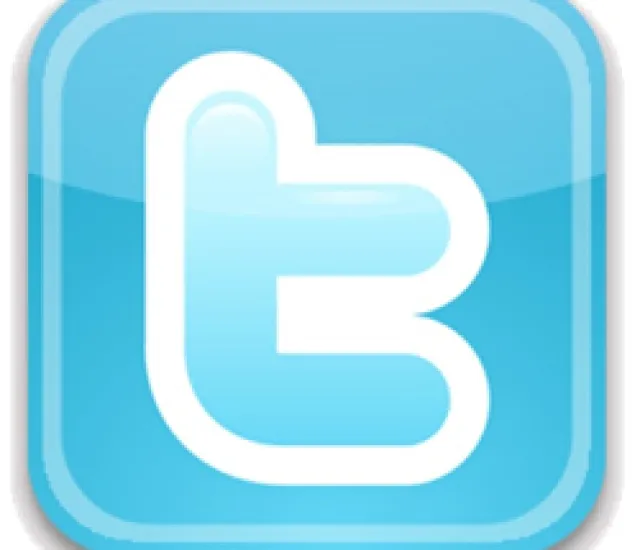
What happens when a 28-year-old British currency trader who previously sold a hair care business teams up with an international trio of informatics professors who publish an academic paper that purportedly unlocks the potential for Twitter to predict stock market moves? They launch Europe's first