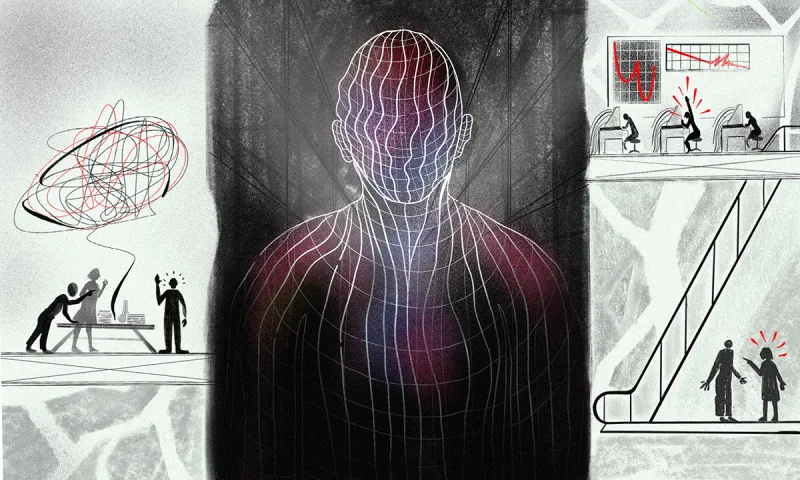
Illustration by Jeremy Leung/II
Move over, smart beta and risk parity: Today’s buzzword is AI. It’s difficult to find a manager that does not claim to be using artificial intelligence to improve its investment process. However, significant obstacles can impede a manager’s adoption of AI — obstacles that threaten to transform not only a manager’s investment processes, but its entire business. Thus many managers’ claims of adoption tend to be more aspirational than genuine. The evidence for these claims typically comes down to one of three things:
The inclusion of a new, nontraditional data set (typically some type of web scraping) into existing traditional, non-AI investment processes; the hiring of a “computer scientist” or “data scientist”; or the simple misappropriation of the term “machine learning” to include traditional quantitative processes.
Such hand waving presents asset allocators with a challenge: separating the counterfeit from the authentic.
As an allocator to AI managers [Leo] and an AI-driven manager [Angelo], we’ve been on both sides of the due diligence process and can attest that the challenge is real. We’ve distilled our perspectives into five simple questions that allocators can use to establish a baseline of authenticity and a framework for further due diligence.
“What problem are you trying to solve, and is it worthy of AI (or could it be solved using traditional quant techniques)?”
First, you need to know if a manager’s claim of AI adoption is related to an investment or non-investment problem. Although AI might help a manager improve compliance oversight and post-trade processing, you are considering hiring the manager because it claims to have some edge that allows it to consistently generate the promised returns. You need to know how this edge is achievable only with AI.
Be ready for a canned answer: “We’re using it to build tools to make our investment process better.” Press: “What types of computational techniques are you building?” Push back on the jargon — RNN, CNN, SVM. Ask the manager to describe, in everyday language, its choice of tools and, importantly, to explain why and how it expects these choices to directly and indirectly improve its investment decision-making.
Our experience shows that you can expect managers to talk less about the tools and more about data. They love to discuss “ingesting alternative data.” Data can certainly provide an edge — but don’t be wowed at the mention of exotic data sets (e.g., a news headlines data set for sarcasm detection — yes, this is a real thing). Ask the managers to delineate the types of data they are using and to explain the nexus between the data and the problem to be solved. How do they procure data? Who validates and manages the data? Also ask about the supporting data architecture. The answers to these questions will help you determine if this AI thing is a one-off (expensive) marketing effort or if there is a genuine commitment.
If there is a plausible use case, then shift to the topic of implementation and integration.
“How did the firm decide to include AI in its investment process?”
Was it board-driven or merely board-approved? Was it championed by the C-suite? The investment team? Anything less than senior management’s full support indicates a tepid commitment.
More important, ask who owns the business decision to shift to AI. Someone — a specific person — must be responsible for the AI project if it is to be successful. Identify that person and, if appropriate, request a call or meeting to verify ownership, competency, and bandwidth.
“What is the status of the AI project?”
This question benchmarks the manager’s commitment at a specific point in time and allows you to inquire about implementation and integration. For example, because you can’t do real AI with just CFAs and MBAs, you should expect the manager to tell you it has made new hires specifically dedicated to the AI project. Get the names and positions of these professionals so you can vet them yourself (e.g., review their publications and patents for relevance and timeliness). Know that in general, predictive analytics requires more than a single scientist; there should be a scientific team with deep knowledge of statistics, mathematics, data engineering, machine learning, software engineering, visualization, and nontraditional data. And because there is a global arms race for this scientific talent, ask the manager how it recruited the team. The response will show if it has adopted HR practices that increase the likelihood of attracting the necessary talent — e.g., the use of contests. (Surprised by this idea? Consider that WorldQuant’s International Quant Championship drew more than 11,000 contestants from 1,000 universities in 80 countries for five full-time positions and 15 internships.)
Extend your line of inquiry. If these individuals are the driving technical force behind the AI effort, what is the manager doing to retain them? The answer will disclose if and how the manager might have changed its compensation structure. Follow up by asking what, if any, policies and procedures have been implemented to ensure the continuity of the AI project (e.g., proper documentation of the technology, adequate security against bad actors), as turnover is inevitable.
Once you know the manager has specialized resources hired and locked down, ask what steps have been taken to ensure this team is being properly integrated into the investment group(s) and the business in general. Unlike traditional investment research, which is often confined to a particular silo, predictive analytics is a team sport. The best work is done through collaboration by cross-disciplinary teams, with the scientific team embedded in those teams, and when the product owner is the business person who will be the direct beneficiary or user of the product (i.e., the portfolio manager). We’ve found that success requires at least one person dedicated to managing this integration. It would be helpful and appropriate to ask to speak with this person.
“What’s your budget for this AI project, and where is the funding coming from?”
AI adoption is expensive; even a modest effort costs millions of dollars per year. Because AI is inherently a long-term project, the manager should provide a budget that is congruent with the problems to be solved. The heart of any AI project is the research agenda, so ask the manager to share it. You don’t need the technical details, but the manager should be able to tell you its R&D plans (including work flow) and how they help solve their specific problem. Also, find out the source of the funding, which could reveal if the manager is making cuts elsewhere in the organization.
“What are the results to date?”
The manager will undoubtedly tell you it is too early to draw any conclusions or point to any results, but trust us: If it tells you it is using AI, it should be able to tell you about AI’s contribution — for why would a manager even mention AI if it didn’t have at least some preliminary positive results? How does the team feel about the results and the progress thus far? What would the manager change, and why? And though no one has ever seen a bad back test, if you are satisfied with the answers so far, now is the time to ask for these pro forma results. (And do not be pushed into signing an NDA to view them.) If the manager has no empirical evidence of its output and the contribution of its models to share, suspect hand waving, not AI.
On this much we agree. However, our respective professional roles have given rise to some differences of opinion.
Leo: Today the generally poor performance of the active management community and the existential threat from “the machines” have managers grasping for a quick fix.
Many seem to think that AI offers that solution.
There are managers for which AI is a natural extension of work they are already doing, for which the staff is already in place and the expertise exists internally. Other managers are convinced that AI — usually in the form of alternative data sets — is table stakes, but don’t have a clear understanding of what that means for their organizations and investment processes. They are more likely than not to find their experiments with AI to be expensive distractions. For this reason, don’t discount managers who are avoiding the hype. A manager that honestly admits that it doesn’t see an application for AI in its process, isn’t really sure where to start, or isn’t willing to spend the time and money to fully investigate the application may be a realist rather than a Luddite.
Allocators are in a more advantageous position, partly because they don’t have to operate in the same competitive environment as asset managers. They can look to experiment with AI in their own processes through internal development and partnerships with managers and research firms. Allocators have the opportunity to see what is and isn’t working in the space and can leverage the expertise and experience of external managers to think about potential projects of their own. As more companies develop data science teams, there may be internal corporate resources that pension plans can use. Endowments and foundations attached to large research organizations or universities are even more advantaged. The good news is that there isn’t the same pressure to solve the problem, and the bar is lower; even small improvements in the investment process can be worth tens of millions of dollars to a large institution. Allocators can watch what happens in the asset management community, leverage internal resources, and put together a thoughtful approach to experimentation and implementation.
Angelo: An AI investment requires a Sisyphean effort. The barriers to success are overwhelming and, to Leo’s point, though many managers could benefit from an improved investment process, many will choose the status quo over transformation.
I also agree that allocators would benefit from incorporating AI into their own investment decision-making. However, they face these same barriers to adoption, especially with regard to funding and resource allocation.
Although Leo’s idea of a partnership with managers is appealing, it is a chimera, built on the illusion of allocator leverage. Examples of genuine knowledge transfer between manager and allocator are rare. (Readers, do not be so gullible as to think that “strategic partnerships” are the remedy. I’ll tackle this charade in a future column.) Any sharing of AI knowledge between manager and client tends to be superficial and limited to white papers, webinars, and marketing decks. The use of AI-related resources within the allocator’s own ecosystem is an innovative and more promising alternative — though within some organizations it requires the CIO to eat a slice or two of humble pie.
Rather than focus on the barriers to adoption or seek an external remedy, I would suggest allocators first look inward to determine if they have the conditions that support adoption.
An allocator might start this introspection by examining its governance structure: Is the board humble enough to admit the shortcomings of traditional active management? Does it generally appreciate the potential advantage AI could provide in the management of its liabilities? Will it genuinely empower the professional staff by giving it the authority (expressed as new key performance indicators) and resources (i.e., a budget) to explore AI adoption in earnest? Without the board’s support, AI adoption in any meaningful way will not be possible.
But before engaging the board, I would caution the professional staff to look in a mirror and ask a simple question: Do we want the board to address the issue of AI adoption knowing that, even with the board’s blessing and a commitment of additional resources, the likely result of this investigation will be failure?