Asked if he’s offended when people trash his investment ideas, Harry Markowitz chuckles like a kindly uncle. The founder of Modern Portfolio Theory replies that he’s in the videoconferencing business. From his San Diego office, he delivers lectures around the globe for $15,000 a pop. Markowitz lets audiences choose from a handful of nontechnical topics — among them, whether his influential blueprint for portfolio construction stopped working during the crash of 2008.
“My business has been brisk in explaining why Modern Portfolio Theory is still correct,” he says.
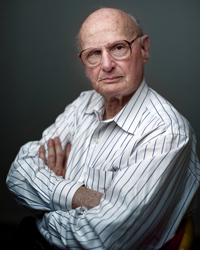
As Markowitz admits, though, MPT has taken plenty of knocks along the way: “For years — maybe almost from the beginning of [Modern] Portfolio Theory — there have been people who’ve been saying, ‘Well, that’s obsolete. We’re going to do something new and better.’”
This chorus has grown louder since 2008. According to critics, diversification offers little protection against markets plunging in lockstep. It may be the right thing to do in normal conditions, they say, but it fails exactly when you need it the most, during times of crisis.
Institutions ostensibly using MPT in 2008 got omelet on their faces. According to the Commonfund Institute of Wilton, Connecticut, and the Washington-based National Association of College and University Business Officers, U.S. college and university endowments lost, on average, 18.7 percent for the year ended June 30, 2009. Many of these funds followed the so-called Yale model of portfolio construction, which advocates diversification through significant exposure to alternative asset classes like hedge funds and private equity. When these illiquid investments stopped throwing off cash — alternatives plummeted, on average, 17.8 percent between July 2008 and June 2009 — endowments had trouble meeting their commitments.
In the end, this problem was more about liquidity than portfolio theory. Correlations head toward 1 in every bear market, says Jeffrey Geller, CIO of New York–based J.P. Morgan Asset Management’s U.S. global multiasset group. But three things combined to make 2008 a different animal: The credit markets all but froze, there was widespread deleveraging, and liquidity rapidly declined.
“People underestimated the risk they had in their portfolio vis-à-vis their demands and requirements for liquidity,” Geller says. “It was felt across all institutional portfolios, but perhaps most across endowments that typically hold larger allocations to alternatives.”
There’s no doubt that markets are far more complex and volatile than they were in 1952. In this changed world, which presents more challenges and more opportunities, MPT could use a makeover just to hold down a job. The recent meltdown was a powerful reminder to stop blindly obeying B-school axioms, including the models of Markowitz and his intellectual descendants. As sound as it may be academically, MPT is vulnerable to big market moves and ripe for misuse. In response, practitioners have built portfolio construction tools that they hope better reflect how markets actually behave. These efforts include fresh takes on optimization, a computer-assisted method of generating portfolios. They also involve making portfolios more resilient to turbulence by building in some recognition that the relationship between risk and return changes over time.
Like many theories, MPT makes a host of simplifying assumptions. One of them is that the market is perfectly liquid. MPT also assumes that there are no transaction costs, that investors can take a position of any size in any security they want and that there’s no herd mentality at work. “Last year all of those assumptions probably got violated at the same time,” says Geller’s colleague Rumi Masih, head of J.P. Morgan Asset Management’s strategic investment advisory group.
That’s reason to be more circumspect about MPT, but not to toss it aside like a quaint relic. With understanding of market dynamics still in its infancy, testing is part of the theory’s growing up. So says Lisa Goldberg, executive director of analytic initiatives and talent at New York–based MSCI Barra, which provides indexes, risk models and portfolio analytics to asset managers and other clients.
“This so-called failed theory has a lot of brilliant elements as well as material that needs revision or rethinking,” argues the Berkeley, California–based Goldberg. “By no means should it all be discarded.”
Much of the debate swirling around MPT concerns optimization. Traditionally, that has meant Markowitz mean-variance optimization, whereby investors generate the most efficient portfolio from a basket of assets. First, they use statistical methods to estimate expected returns, volatilities and covariances (that is, how the assets will move in relation to one another during a certain period).
All of this information gets plugged into a piece of software called an optimizer. The optimizer then sifts through every possible combination of assets and produces a graph showing a curve called an efficient frontier. Ranged along it are a series of optimal portfolios, from the lowest risk and return to the highest.
While the benefits of diversification are tough to dismiss, mean-variance optimization doesn’t stand up so well, for a couple of reasons. It can produce questionable portfolios, and it makes no allowance for fat tails — returns that fall far outside historical norms. Also known as “black swans,” these unexpected and dramatic price changes can ripple through the entire system, as they did in 2008.
For some practitioners, relying on MPT is simply too dangerous. Svetlozar (Zari) Rachev — chief scientist at FinAnalytica, a risk management and portfolio construction consulting firm based in New York, London and Sofia, Bulgaria — compares it to living beneath an avalanche-prone mountain. “The correlations that are embedded as the main assumption — the normality of returns — are telling you the chance of an avalanche affecting everybody in the village below is zero,” he says.
Also, according to Rachev, Markowitz never intended to create a universal model that works for any portfolio. “He did MPT for one type of problem: quiet markets, constant volatility, constant correlation,” Rachev says. “The world now is not such. We need different solutions.”
Then again, people should have known better than to apply MPT naively. In the lead-up to 2008, they took on too much risk, foolishly betting that the avalanche would never come. MPT “did not fail,” Markowitz says. “Some financial advisers, but certainly some people on the sell side, sold things without a proper analysis of how they affected the portfolio as a whole.”
Markowitz hangs his defense of MPT on the simplified version of the theory laid out in 1964 by longtime Stanford University finance professor William Sharpe, who at the time was teaching at the University of Washington (see timeline, page 68). Now known as the capital asset pricing model, or CAPM, Sharpe’s “one-factor” theory assumes that all assets in a portfolio share systematic, or market, risk. The source of beta returns, this common risk factor is impossible to diversify away. Each security, however, also has an unsystematic, or idiosyncratic, risk, which generates alpha. Because the returns on different assets don’t tend to line up exactly, you can shrink that risk through diversification.
But in a crisis, market risk swamps idiosyncratic risk. As a result, Markowitz explains, everyone moves downward — but not the same distance. Just like MPT says, the more beta you have, the farther you fall.
Besides, diversification actually worked in 2008. Roger Ibbotson, a finance professor at the Yale School of Management, notes that high-quality bonds were up while equity markets fell 40 percent overall. Although beta, or volatility, risks are the key drivers of stock returns, Ibbotson adds, the role of liquidity has often been ignored. “That liquidity component of what affects returns is every bit as important as the risk component,” says Ibbotson, who is also chairman and CIO of Milford, Connecticut–based Zebra Capital Management.
Markowitz says investors should know where their beta puts them on the efficient frontier. But he admits that MPT doesn’t make sophisticated assumptions about probability distributions — a big problem for highly leveraged investments that get marked to market daily. “There are ways of acting where you’re exposing yourself to model risk in a very major way,” says Markowitz.
The solution is to make estimates that reflect future uncertainty. In addition to being an adjunct professor of finance at the University of California, San Diego’s Rady School of Management, Markowitz is co-founder and chief architect of GuidedChoice, a Los Gatos, California–based 401(k) adviser. He says GuidedChoice favors volatility and return estimates that are, respectively, at least as high as and slightly lower than the historical average. This cautious outlook encourages clients to settle on a portfolio of, say, 60 percent stocks and 40 percent bonds, rather than leveraging highly on dubious assumptions.
You could also build a better optimizer. One firm that claims to have done so is Cambridge, Massachusetts–based Windham Capital Management, which developed full-scale optimization with a division of Boston’s State Street Global Markets. According to its creators, this technique is much more sensitive to extreme events than mean-variance optimization.
Windham president and CEO Mark Kritzman compares commonly used optimization methods to having one set of clothes based on the average temperature in Boston. “I like to tweak people — I say, ‘The only thing wrong with diversification is that it’s never been tried.’”
In his 2006 book, The Poker Face of Wall Street, Aaron Brown makes a strong case that gambling is intrinsic to financial markets. Brown should know — the veteran quant played poker semiprofessionally as a student in the 1970s and ’80s. Today he’s a risk manager at Greenwich, Connecticut–based hedge fund and asset management firm AQR Capital Management. Brown doesn’t think poker players make particularly good portfolio managers, but he says there are mathematical similarities between his favorite game and portfolio construction.
In poker, playing only your strong hand can be disastrous, Brown explains. But playing too many weak hands means throwing money away. “Similarly, as a portfolio manager, if you go only with the best ideas, you’re not diversified enough,” says Brown, who was an executive director in risk methodology at Morgan Stanley in New York before he joined AQR in 2007. “But if you strive for maximum diversification, you get a lot of bad ideas in there.”
Academically speaking, Brown says, the basic idea of MPT is unassailable: You identify asset classes and try to estimate what return you can expect for a certain amount of risk. Then, rather than consider each asset class individually, you build a diversified portfolio with an acceptable risk-return trade-off. “That’s never been challenged, and I don’t think it ever will be,” says Brown, who has an MBA in finance and statistics from Markowitz’s alma mater, the University of Chicago.
But in practice, MPT sets several traps that can lead investors astray, Brown adds. First, it may blind them to speculative bubbles by giving the impression that an asset is equally risky at its all-time high and its all-time low prices. It also pushes unsophisticated users toward the idea that the future will be like the past when it comes to deviations from normal returns. In addition, MPT defines risk in terms of price movement, making an implicit assumption that everything can be bought and sold at a given price whenever an investor chooses.
“If you can’t get your money out of an investment, it doesn’t really matter what the mark is,” Brown says. “It’s not a true mark, because you can’t buy and sell at that price.”
Besides creating products that reflect its views on what makes an optimal portfolio, Brown’s firm helps institutional and individual clients build their own. David Kabiller, a founding principal and head of client strategies at AQR, which has $24.5 billion under management, says the discussion is different every time. Still, most large institutions have a limited appetite for illiquid private equity, Kabiller asserts. “And there are only so many hedge funds and so much skill out there,” he says. “If institutions are going to meet their investment objectives, it’s going to be more heavily influenced by how they structure and allocate to core betas.”
With that in mind, AQR offers its $2.5 billion Global Risk Premium product, which consists entirely of liquid securities. Brown says Global Risk Premium’s two funds invest in a variety of asset classes, including equity and bond indexes, commodities and Treasury Inflation-Protected Securities (TIPS), because doing so provides a lower and more predictable risk for a given level of expected return. But investing so broadly has two big potential pitfalls. One is the temptation to use excessive leverage; the other is model risk when making guesses about volatilities and correlations.
AQR guards against those potential perils with leverage caps and drawdown and exposure controls. “These portfolios require more-attentive risk management,” Brown says. “But we feel that the reward in terms of lowering risk — and especially lowering tail risk — is worth it.”
AQR starts by assuming that each asset class has its own independent risk. Mindful that assets tend to correlate, especially during crises, it seeks the broadest possible exposure. Right now, Brown says, there are very high correlations between equities and commodities, equities and credit, and interest rates and foreign exchange. Although those assets don’t yield much diversification today, he says, you’re better off buying them because they may diverge. And even if they end up being 100 percent correlated, it doesn’t hurt to split your money.
But if correlations are extremely high, sticking with long-term target allocations is too risky. Brown says today’s odd combination of low volatilities and high correlations makes many investments look safer than they are, but volatility can spike quickly.
“It’s a very scary market environment, almost scarier than a volatile environment like the fall of ’08,” he explains. “It’s too quiet out there.”
Richard Michaud, co-founder, president and CIO of Boston’s New Frontier Advisors, began questioning mean-variance optimization almost four decades ago. After receiving his Ph.D. in mathematics and statistics from Boston University in 1971, Michaud went to work as a senior research analyst at the Boston Co. investment firm. One of his first assignments: use mean-variance optimization to research the development of a European country fund. Michaud had to get tapes of the Markowitz optimization programs and run them on Boston Co.’s mainframe computers.
Proud of the optimized portfolio he produced, Michaud showed it to the firm’s research director, who wasn’t impressed. “‘What is this, Dick? We need to invest 34 percent in Austria?’” Michaud remembers him saying. “As he understood, that was a crazy investment result, but it was also the correct result from the optimization.”
Intrigued, Michaud talked to his quant friends and learned that they’d had similar experiences with optimizers. Drawn to academia because it allowed him to study such problems and broaden his financial knowledge, he left Boston Co. in 1977 to teach advanced portfolio theory at Boston University. Michaud later switched back to finance, holding posts that included head of equity analytics at New York–based Merrill Lynch & Co. before launching institutional research and investment advisory shop New Frontier in 1999.
Throughout his career Michaud kept thinking and writing about optimization. He began collaborating with his son, Robert, who is managing director of research and development at New Frontier. The pair developed and patented a portfolio optimization process called Resampled Efficiency.
The elder Michaud says the trouble with traditional optimizers is that they assume the information you feed them is perfect — to at least 16 decimal places. If you’re performing a scientific task like landing a rocket on the moon, that level of precision is the bare minimum. “But in finance it’s absolute nonsense,” Michaud says. “Sixteen decimal places of accuracy for whether stocks are going to beat bonds or not? Investors are more than happy just to get the sign right.”
Besides, users often don’t like the portfolio their optimizer coughs up, so they change the inputs. “Eventually, you do what’s called a why-bother optimization,” says Robert Michaud, who has MS degrees in mathematics from Boston University and finance from the University of California, Los Angeles. “You’ve tortured the optimizer to give you what you knew in your heart was right anyway.”
According to the younger Michaud, Resampled Efficiency builds on Markowitz’s good work. But rather than forecasting risk and return exactly, it allows some room for error. Using a statistical method called a Monte Carlo simulation, the optimizer generates thousands of possible market scenarios. It then recommends investing in the portfolio that performs the best across all of them. “That results in something a little more tolerant to markets not working out quite the way you were expecting them to,” Robert Michaud says.
There’s evidence that Resampled Efficiency works. Besides selling its optimization software, New Frontier manages about $1 billion in 15 Global Strategic ETF model portfolio strategies offered through Pleasant Hill, California–based Genworth Financial Wealth Management. Designed to be fixed-risk core investments for long-term institutional investors, these products run from 20 to 100 percent in equities. Optimized, rebalanced and managed using Resampled Efficiency, they contain a mix of domestic equities, fixed income, real estate and international exchange-traded funds.
With 20 percent in equities, New Frontier’s most conservative fund is the $64 million Global Income Portfolio. Since its October 2004 inception, it has posted an annualized return of 4.1 percent. In 2008 the fund was the third-best performer among ETFs ranked by iShares, a division of New York–based BlackRock. Its –4.4 percent return far outstripped the Standard & Poor’s 500 index, which finished the year down more than 37 percent.
Resampled Efficiency has also been put to the test by Markowitz, who is a friend of the Michauds. In a 2003 paper in the Journal of Investment Management, Markowitz and Montclair State University finance professor Nilufer Usmen tried to answer the question of whether a better optimizer trumps better information. Setting up a refereed duel between two simulated players, they structured some raw historical return data and plugged it into a traditional optimizer, then ran the same data through the Michaud optimizer.
Markowitz and Usmen set out to beat Resampled Efficiency. But to their surprise, the Michaud optimizer won all 30 tests. “I’m not sure why his process works so well, and I don’t use it,” Markowitz says.
In Richard Michaud’s opinion, the serious innovations in portfolio theory that lie ahead will have mathematical proofs behind them. He doesn’t put much stock in the various statistical modeling techniques that have recently become finance buzzwords. “There are lots of people coming out with fancy terms like ‘copulas’ and ‘extreme events’ and all kinds of alternative ways of looking at statistics,” he says. “Most of them are not well informed at all.”
As AQR’s Brown points out, an optimizer is only as good as its input data. If you knew the covariance of returns for the next five years, even a basic one would suffice. And if your estimate is way off, no optimizer will save you.
No fan of off-the-shelf solutions, Brown says optimization calls for sound judgment from experts in individual asset classes. The more optimized a portfolio is, the more model risk it has, he adds. Brown says that isn’t a bad thing, provided you monitor the portfolio closely and have a contingency plan. Just as optimization can improve portfolio performance enough to justify the model risk, skipping the process entirely is foolish. “You need some optimization, but you want to be careful how much you use,” Brown says. “It’s a dangerous thing.”
The same goes for too much accuracy. Although AQR strives for precise portfolio weights, it knows when to stop. “Any optimization that is telling you it really matters a lot whether you’re 4 or 5 percent in something is just not very useful,” Brown says. “A robust optimization program will give you weights that if you change them a little bit, it won’t change the result very much.”
As far as Mark Kritzman is concerned, there’s nothing wrong with MPT. If investors choose to apply the theory naively, that’s their problem. “You start out with a model that uses simplifying assumptions, and then you relax those assumptions and build in complexity,” says the Windham CEO. “Some people built in more complexity than others.”
Contrary to popular belief, Kritzman explains, MPT doesn’t exhort investors to extrapolate historical returns, volatilities and correlations. Instead, they must come up with their best future estimates for a chosen horizon and diversify accordingly. Investors who did so suffered less than others in 2008, Kritzman contends.
To illustrate his point, he offers this example. Imagine that before the crisis hit, you used historical data to estimate the value at risk (VaR) over a five-year horizon of a traditional portfolio invested 60 percent in equities and 40 percent in bonds. Paying attention only to the distribution of outcomes at the end of the period, you’d have pegged the portfolio’s worst year in a century at a 9 percent loss.
For a result that bears a much closer resemblance to reality, Kritzman says to ditch average correlations in favor of those that have prevailed during turbulent markets, when losses are more likely. This approach would have given you a worst-case number of –25 percent, the loss suffered by a typical 60-40 portfolio in 2008.
“You would have had a vastly different assessment of the portfolio’s exposure to loss, and it would have been pretty much in line with what happened,” he says.
Kritzman would also like you to consider this fact: When U.S. and non-U.S. stocks enjoy returns one standard deviation above average, which is typical of a strong bull market, the correlation of their returns is –17 percent. When those returns are one standard deviation below average, the correlation is 76 percent.
That tells you two things, Kritzman says. First, the average correlation is almost meaningless. Second, investors are getting the opposite of what they want: diversification when their portfolio’s main growth engine does well and unification when it does poorly.
Kritzman, who teaches financial engineering at the Massachusetts Institute of Technology’s Sloan School of Management, says it’s important to estimate loss exposure by thinking of returns as coming from multiple risk regimes. This method of building portfolios that withstand market turbulence is nothing new, he stresses.
In many cases, Kritzman says, mean-variance optimization works just fine. But sometimes returns aren’t normally distributed because of regime shifts or fat tails. Also, on its own, mean variance is lousy at approximating investor preferences. For example, depending on whether returns are above or below a certain threshold, a hedge fund may dramatically change its attitude toward risk.
Kritzman, whose firm offers investment, technology and advisory services, says full-scale optimization is the best alternative. He developed this approach with Sébastien Page, head of the portfolio and risk management group at State Street Associates, where Kritzman is a founding partner. Windham, State Street Global Markets and Cambridge-based FDO Partners formed the jointly owned company in 1999. Meanwhile, most of Windham’s $30 billion in assets is currency run in collaboration with SSGM.
Whereas mean-variance optimization looks at summaries of returns, Kritzman says, full-scale optimization considers all features of the data. It takes into account every single return in an asset’s history — and even in the theoretical distribution — and zeroes in on the portfolio that yields the best outcome. It also factors in any investor preferences that can be turned into equations.
This process is more sensitive to downdrafts than mean-variance optimization, which doesn’t distinguish between upward and downward moves, says Kritzman, who holds an MBA from New York University. “Full-scale recognizes that investors are much more averse to downside deviations and don’t mind upside deviations,” he adds.
In fact, Markowitz introduced the idea of mean semivariance, which measures downside risk, in his 1959 book, Portfolio Selection: Efficient Diversification of Investments. But he says he uses his original optimization method because it’s simpler. Markowitz explains that mean semivariance calls for using historical returns or generating hypothetical histories. “If you do that, you have to pick probability distributions,” he says. “Mean semivariance is more complicated to use and takes more estimation, so I have not been a big proponent of it.”
Kritzman notes that two causes of market turbulence are exogenous shocks and crowded trades. But regardless of the cause, turbulence always has two main features: The ratio of returns to risk is much lower, and the turbulence is very persistent. Kritzman compares it to in-flight turbulence, which lingers until an airplane finds a new altitude or passes through the weather system. “The turbulence may arise unexpectedly, but once it begins, it takes time for investors to digest and react to what’s going on,” he says. “So it’s pretty likely that it’s going to be around for a while.”
Turbulence is not just about volatility, State Street’s Page stresses; it’s also about how assets interact with one another. If turbulence is redefined that way, he says, measuring unusual returns across different assets yields a very different picture. “Sometimes volatility will be low but you start to see things moving in unusual ways, and that can be a predictor of future volatility.”
As a navigational aid, State Street Associates has created an index that quantifies turbulence for any given trading day. Page says the first step is to use this turbulence index to identify periods in history when risky strategies have underperformed. Then, because crises are unpredictable, investors must determine whether turbulence will be persistent enough that they can scale back their exposure to these strategies after it hits.
This kind of dynamic, or tactical, asset allocation works for some investors, but others will simply choose to build a portfolio that is resilient to turbulence. “When you think of the turbulence index, you can think of both dynamic and static, and it depends on investor objectives,” Page says.
J.P. Morgan’s Masih says his group is studying the idea that returns shift according to different economic and financial regimes. For him, the first question to answer is what trigger point will allow migration from one regime to another. The second is what return behavior is commensurate to each regime, based on historical evidence. “Question No. 3 is, What’s the asset-class ranking that I can use to aid my asset allocation decision, given that behavior?” Masih says.
Taking this approach puts less weight on traditional asset allocation and more on behavioral relationships. Instead of trying to forecast the correlation of two assets, the smart thing to do is ask what causes that correlation, Masih says: “These are much more interesting questions that we can explore, which will add insight to the investor’s problem set, rather than ‘Let’s figure out another fancy optimization routine.’”
AQR’s Brown also tends to be skeptical of products billed as improvements on MPT. They’re often simple ideas in elaborate packaging, he says. “My general belief is that there are simple ways to do these things,” Brown explains. “You don’t need to have a whole new approach.”
Still, finance has changed considerably since Markowitz first floated his theory. Back then, information was hard to come by, and the only asset classes were stocks, bonds and cash. Brown says there’s much better data now, as well as the ability to cover far more of the market portfolio. He guesses that when Markowitz was writing in the early 1950s, 30 percent of risk was incorporated into the market. Today there are liquid investments that give access to upward of 80 percent of risk — a huge advantage.
But whereas investors once built portfolios from stocks that all traded in the same market, they’re now up against foreign exchange, swaps, futures and securities with vastly different timescales. “On a practical level, it’s much more difficult, but a big part of the reason is that the opportunities are so much better,” Brown says.
Markowitz agrees that the intervening years have transformed the world, but he points out that investors still must deal with uncertainty. “The details are different, but the principles are the same,” he says. “The laws of probability have not changed.”
As for MPT, Markowitz contends that each market meltdown has delivered new converts. “Maybe the effect of the crisis will be that thoughtful people — the people who will listen — will go back and understand the basic assumptions of portfolio theory, rather than just listening to sell-side salesmen telling them how it has to work.”
Having changed the world himself with an enduring idea, Markowitz has no shortage of listeners waiting to hear him explain why MPT still matters. Whether his successors will enjoy the same returns is something no model can predict.